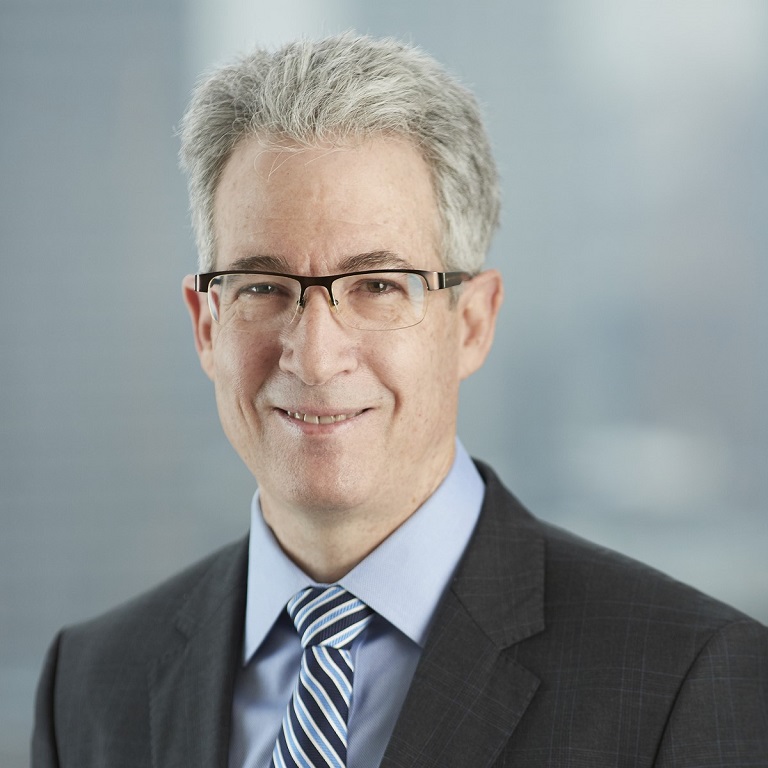
Orrie Dinstein
Global Chief Privacy Officer, Marsh McLennan
-
United States
Keeping a "human in the loop" (HITL) is commonly cited as a strategy to mitigate against artificial intelligence (AI) risks. In fact, in some jurisdictions, this is a legal requirement.[1] This approach is based on the premise that human oversight can mitigate against inevitable technological errors generated by AI. Yet, humans are fallible, inherently biased, and can compound technological errors produced by AI instead of mitigating them. In this article, we explain why prescribing a HITL solution can be overly simplistic as its effectiveness greatly depends on defining the loop, articulating the underlying principle driving the desire for a HITL in order to pick the right human, and having a process to eliminate bias. Without clearly defining these elements, the HITL is doomed to fail to achieve its intended goal.
The term “HITL” is used in varied ways in the AI sector, at times referring to human oversight applied to certain segments of the AI development process (e.g., human oversight applied to model training) and at other times referring to the AI’s operational phase, during which end-users are interacting with the model (e.g., human oversight applied to a customer service chatbot). The former is defined by governance and human supervision over an overall AI system, ensuring it does not cause harm or “go rogue.” The latter is focused on the operational phase where there is a strong desire to mitigate against AI system autonomy or auto-decisioning risks. The idea behind this operational approach is to incorporate real-time, ongoing human monitoring and intervention to help prevent the AI system from making erroneous decisions.
Various legislative and regulatory regimes addressing AI, such as the EU’s AI Act, have introduced the HITL concept such that it applies both to the AI developmental process as well as to the operational ones. In this article we will focus solely on the operational application of the HITL principle.
In the operational context, the "loop" refers to the scope of the AI system’s operation that requires risk mitigation. While there can be several loops in practice, their number is likely fairly limited as the focus here is on mitigating risks associated with decisions rendered by the AI system. Properly defining the loop is important because not all loops require a HITL. In fact, for some loops, a HITL may be counterproductive and cause more errors. To decide what loops are the right ones to apply a HITL, organisations can ask a simple question – where in the system lifecycle is the AI being used to make consequential decisions, such as those with financial, legal, or health-related outcomes? Where such a use exists, there is a risk of autonomous activity that may erroneously render consequential outcomes and a HITL should probably be applied.
Take the example of an organisation that is finetuning pre-trained models (e.g., using platforms like Azure OpenAI) with proprietary data to adapt the model for use in a customer service chatbot. This allows providing customers with faster, personalised interactions with improved availability. In this context, while various loops that require risk mitigation exist (e.g., the phase where the model is trained on the relevant questions and responses, the loop where the model is integrated within the enterprise’s IT infrastructure, and the phase where the chatbot interacts in real-time with customers), the only operational loop in which there is a risk of a material decision being made by AI is the loop where the chatbot interacts in real-time with customers. Even in such cases, organisations should evaluate whether the interaction is significant enough to require a HITL. For example, relatively minor interactions (e.g., where to find a form) may not require HITL and instead just periodic human oversight, whereas more significant interactions (e.g., approval for a reimbursement) may require HITL.
On the other hand, some AI systems can be used in cases without consequential decision-making. For example, an AI chatbot whose function is to help users find forms or content on website is designed to automate repetitive, rule-based tasks to increase efficiency and accuracy in directing users to the appropriate information, reducing the potential for human error. In the event of an error, a user could easily be redirected to the appropriate page. Adding a HITL in this context would negate any gained benefit. Similarly, adding ongoing, continuous human intervention in an AI model that is enhancing images in photographs based on predefined algorithms according to objective criteria (e.g., noise reduction, color correction, etc.) is likely to cause more errors due to human fallibility.
As such, it is important for decision-makers to focus on the different types of AI and the various use-cases, providing clarity on situations where a HITL adds value as opposed to scenarios where it may result in harm.
The phrase "human in the loop" is often used without qualifying who or rather what expertise is required. Is this meant to be someone who understands the underlying business process in which AI is being used? Or someone who understands the AI tool and where it might fail? Does it require someone with decision-making authority to override the tool or merely someone who can observe and validate the results or report on a perceived failure? These distinctions are critical to designing the right HITL safety mechanism.
What this means in practice is that in order to decide where and how a HITL adds value, organisations need to clearly define the underlying principle driving the desire for a HITL prior to assigning the “human” to provide oversight. For example, if the driver is transparency, domain knowledge is crucial as the human needs to understand how the AI system works. If the desire is accuracy, the human needs to understand the subject matter being handled by the AI. If the desire is to meet legal or regulatory obligations (many laws have restrictions on auto-decision making) then the human needs to be versed in these rules. And if the desire is to ensure “fairness,” the organisation should define the parameters of fairness and how it is measured. Unless the goals of oversight are defined in advance, assigning a human to act as a HITL could be a recipe for trouble.
Applying this rationale to the case of a loop involving a customer-facing chatbot, the underlying principle likely centers around accuracy in servicing customers. Thus, the “human” expertise required likely consists of seasoned customer service representatives equipped with the authority to intervene in specified circumstances (e.g., decisions involving refunds), to ensure appropriate outcomes for the customer. In a different “loop” that involves an AI tool helping screen job resumes, the underlying principles likely involve fairness and transparency, in which case the human expertise should likely consist recruiting experience. A single organisation will likely use multiple AI tools for different use cases and will therefore need to define multiple “loops” with respective human expertise providing oversight in each defined use case.
In discussing the need for a HITL, the introduction of a human element is often viewed as a way to mitigate against the risks of model bias and discriminatory outcomes by AI. But human involvement is not in-and-of-itself a sufficient safeguard against the risks of AI-associated bias and discrimination – after all, every human is biased, and we all bring our biases to our jobs. Sometimes, humans may even exhibit a bias towards deferring to an AI system and hesitate to challenge its outputs, undermining the very objective of human oversight. Whether the “human” providing oversight is an individual or a larger team, human oversight without guiding principles and a process to define and evaluate bias may amount to merely swapping out machine bias with human bias. Bias can never be reduced to zero – it is inherent to humans, and therefore to AI systems designed by humans. The only way to mitigate bias is by clarifying what kinds of bias are present, and establishing to what degree they are tolerable. This requires clearly defining target outcome metrics as proxies of bias, and guiding principles paired with meticulous assessment of the input and output model data to see how closely they are aligned with the respective metrics and principles. This process should be iterative, consisting of periodic re-evaluation of the baseline model data that may with time no longer appropriately reflect how the world has evolved. Given both AI and humans are inherently biased, such a process could benefit from a symbiotic relationship between AI and humans, where humans evaluate potential biases in AI systems, and AI systems in turn help surface potential human blind spots and biases.
In conclusion, requiring human oversight for AI systems is not in-and-of-itself a panacea to mitigating against AI risks and it can, at best, lead to a false sense of security and, at worst, compound the risks. Solving AI risks with a HITL requires:
[1] For example, the EU AI Act (Article 14) requires high risk systems be designed such that "natural persons can oversee their functioning, ensure that they are used as intended and that in their impacts are addressed over the system’s lifecycle."
Global Chief Privacy Officer, Marsh McLennan
United States