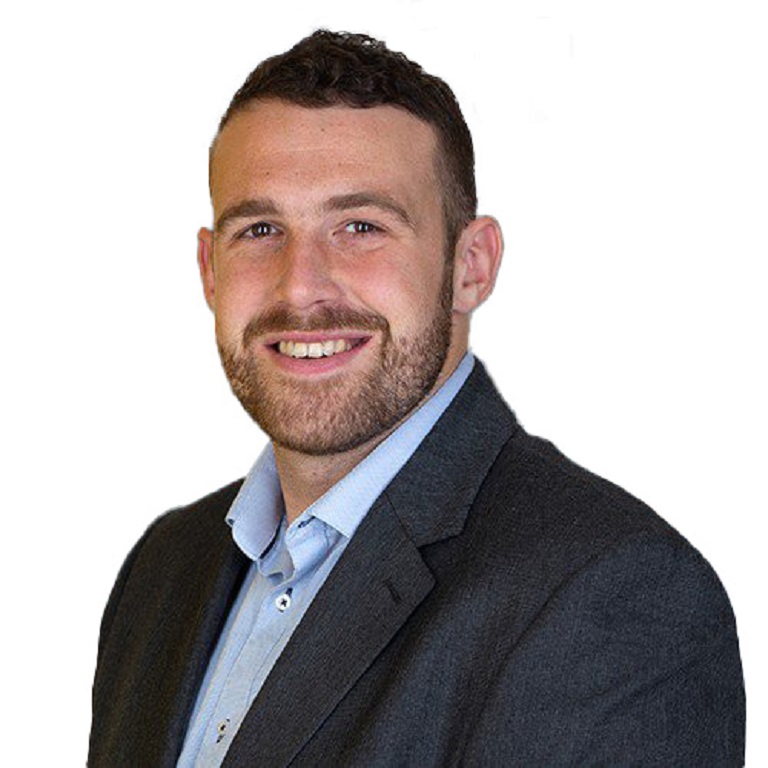
Jason Mitchell
Actuary, Vice President, Marsh Advisory
-
United Kingdom
From airlines to airports and manufacturers — there is huge potential for companies across the aviation sector to use modeling and risk analytics to maximize the value of their insurance programs. These techniques can improve organizations’ understanding of risk exposure and, crucially, loss volatility, plus also provide a cost-benefit assessment of different insurance programs with the data to design optimal risk financing strategies.
These were among the insights shared at the analytics and modeling workshop at Marsh’s inaugural aviation summit. Experts discussed how analytics enable companies to gain greater control of their insurance program, make more informed decisions on which risks to retain and those to insure, and identify risk correlations and opportunities for diversification in advanced program structures.
Analytics help a company to evolve from being a buyer of insurance to a transferor of risk. Rather than starting engagement with the insurance market from an existing, expiring insurance structure as a base, a transferor of risk can take greater control of their insurance program by internally pre-qualifying a range of insurance strategies through analytical work before approaching insurers. They can then seek to transfer a more consciously defined amount of risk with a view of the associated volatility and potential financial impact, if that risk materializes. The analytical findings and data give the insureds a better grasp of the level of risk they could benefit from retaining and where insurance can add most value.
Companies across the aviation sector, for example, typically expect losses such as trips, spills, and baggage claims that are usually smaller in value, occur more frequently, and are hence more predictable. Company-specific, “ground-up” loss models use an insured’s own historic loss data for these types of losses, to generate projections that best reflect the company’s own risk/loss profile. Some companies will decide to retain these more expected losses and use insurance capacity for more costly, less frequent, and less predictable events (such as a total hull loss) that fall outside their insurable risk appetite. However, it is worth noting that (re)insurers usually model the global aircraft fleet and then allocate that in some way to particular insureds. Such models are, therefore, typically skewed more towards catastrophic exposures and scenarios and, as such, these “top-down” models are more likely to positively adapt to reflect a specific fleet’s loss experience, exposures, and deductible structures, if the potential dollar swap of the attritional losses is managed more effectively.
Companies with a comprehensive understanding of their potential loss volatility can design a risk financing strategy better aligned to their risk tolerance and risk appetite. One approach to transferring risk entails companies deciding on the level of volatility they wish to transfer and the range of price they are willing to pay to transfer that exposure. Operating within these “guide rails,” and combined with other non-analytical placement methods, it is possible to create a bespoke program that can assist to withstand shock losses and help to somewhat offset market cycles.
Predictive analytics allow companies to set a longer-term risk financing strategy, and multi-year plans with clear guide rails also tend to avoid the chasing of short-term benefits at the sacrifice of long-term objectives.
“Economic cost of risk” (ECOR) builds on the more widely recognized total cost of risk metric by further taking into account fluctuations in losses from year to year. Once organizations have quantified their economic cost of risk, they can see the cost of both more known risk and the cost of absorbing volatility over a specified period. Average annual retained losses can be calculated by some companies internally, but volatility can be difficult to quantify without more advanced statistical methods. Armed with an economic cost of risk value for the company’s current insurance program and a set of alternative strategies, risk managers can directly compare the relative economic value of different insurance strategies to inform and demonstrate their decision-making.
With regard to advanced risk financing structures, such as captive self-insurance and potentially captive reinsurance, analytics and modeling can help companies set retention/transfer stop-loss levels according to their risk appetite, and evidence risk diversification across insurance classes or across years. These tools can also enable a company to better determine the probability of a loss being paid by a given reinsurance layer and explore profit-sharing opportunities with (re)insurers under certain structures. For parametric insurance, models can identify correlations between external and client data sets and assist in assessing appropriate indemnification amounts.
There are substantial financial and risk management benefits to having the right insurance policy in place for your company. Risk analytics and modeling provide an enhanced understanding of your risk exposure and potential loss volatility and ultimately can help you to develop your insurance program into a valuable, strategic asset.
It is also important to note that price is only one measure of the cost of an insurance program. Without analytics, an insured cannot always see how different insurance program structures might impact their exposure to volatility in retained loss costs. Working with a qualified insurance consulting partner to help you quantify your economic cost of risk, can better position you to compare the suitability of various insurance programs for your company. Deploying a high level of analytics and modeling can also allow you to develop strategic insurer engagement plans designed to help you get the best out of your insurance purchase and financing strategy.
The most appropriate insurance program for an insured is not necessarily the cheapest — price is just one metric. Analytics help an insured to see how much risk — in financial terms — is being transferred to the market for the premium paid. Often, there are opportunities for insureds to invest in their insurance program to improve its quality. For example, a program that results in the payment of losses in a timely manner may represent better value for money in the long term — even if it costs more — than a cheaper alternative where losses are paid hundreds of days after the loss event.
Yes, fluctuation in interest rates can impact a company’s cost of risk, depending on how cost of risk is being calculated. If your cost of risk factors in the cost of deploying your own capital to fund volatility you could have otherwise insured, then interest rates most certainly can impact your cost of capital. It will also impact an insurer’s cost of capital which is inherently linked to their profitability and market pricing.
This is why we recommend that insureds should conduct an analytical review of their insurance programs at least every other year or every third year, to assess whether the insured’s program is appropriate for the current economic circumstances.
Insureds should ask whether the model and its data are appropriate for their business.
In general, aviation is a heavily data-driven industry that tends to learn from past events and previous trends. The sector benefits from having access to data from past events and numerous sources that can be repurposed and adjusted.
The more volatile a risk, the more simulations will be run on models and assumptions may be validated with the client.
Parametric products that pay out based on predefined parameters or indices rather than traditional loss assessment methods are used in the aviation sector. They are predominantly for business interruption exposures and so sit parallel to the aviation insurance programs, as part of clients’ overall insurance programs. Business interruptions arising from ash cloud or hailstorm events, for example, could potentially be well suited for cover via a parametric. Executing a parametric deal usually relies on having the right mix of client, underwriter, data, and insurance market conditions at a given point in time. There is a general expectation that these types of structures will become more prevalent in the aviation sector. Analytics and modeling help to identify correlations between external and client data sets and assist in assessing indemnification amounts for parametric insurance structures.
Actuary, Vice President, Marsh Advisory
United Kingdom
Analytics Development Leader, Senior Vice President, Marsh Advisory
United Kingdom
Vice President, Risk Management Practice
United Kingdom